Researchers
Aayush Vikram Singh
Jayesh Kartik
Shivangi Sharma
Faculty Advisors
Dr. Cristian Homescu
Abstract
This research paper identifies the distinct patterns that may come from market crashed and bubble patterns, which are much different from the normal market condition. These patterns are called "regimes" and are crucial in strategic asset allocation within portfolio management. The research uses advanced statistical models like the Markov Switching Model (MSM), Hidden Markov Model (HMM), and Gaussian Mixture Model (GMM) to be able to differentiate these market regimes. The goal is to create trading strategies tailored to the current market regime, which can then enhance the performance of investment portfolios.
Introduction
The research shows how market regimes might affect investments, especially during market crashes and booms. These regimes have distinct pattern which impact asset performance, emphasizing the importance of adaptive forecasting models and investment methods. The research focuses on regime-based asset allocation, while trying to improve portfolio performance by responding to regime transitions. This technique uses data from a variety of asset types, and aims to achieve better long-term results than static solutions. The research investigates the dynamics of market regimes and their importance in portfolio management, emphasizing the classification of asset performance into separate periods with similar behavioral characteristics.
Research Objective
The research uses modern machine learning and statistical methods, such as the Markov Switching Model (MSM), Hidden Markov Model (HMM), and Gaussian Mixture Model (GMM), to detect and then forecast changes in specific market circumstances. It identifies market regimes, computes transition probabilities, and discovers preconditions that indicate regime transitions. The research adds regime-based asset allocation techniques into the portfolio optimization to improve long-term investment performance. To understand regime dependencies, the technique includes an investigation of many asset classes along with economic indices, such as CPI and unemployment rates. This strategy straightens out market dynamics, assisting investment managers to make better decisions and predictions.
Data and Methodologies
This research data is from 2004 to 2023, which includes a wide range of asset types such as commodities, hedge funds, equities, and bonds. The asset classes were selected with a focus on their relevance to specific subgroups, broad availability, liquidity, and representation of market regimes. Along with these asset classes, the study simulates regime dependency using data from the CPI and unemployment rates, improving knowledge of how bigger economic indices influence market trends.
The strategies used in this study are broad and robust, including multivariate regression, time-series modeling, random forest models, and Markov regime-switching algorithms. Notably, the multivariate regression analysis, shown in Figure 1, examines the influence of various economic data on market indices. This method is critical for determining how changes in the CPI, unemployment rates, and other economic indicators link with fluctuations in market regimes. The datasets used in this study were chosen precisely because of their statistical features, which make modeling easier and more trustworthy than analyzing individual stocks or bonds. This comprehensive approach enables a thorough grasp of market dynamics and the numerous elements that influence changes across market regimes.
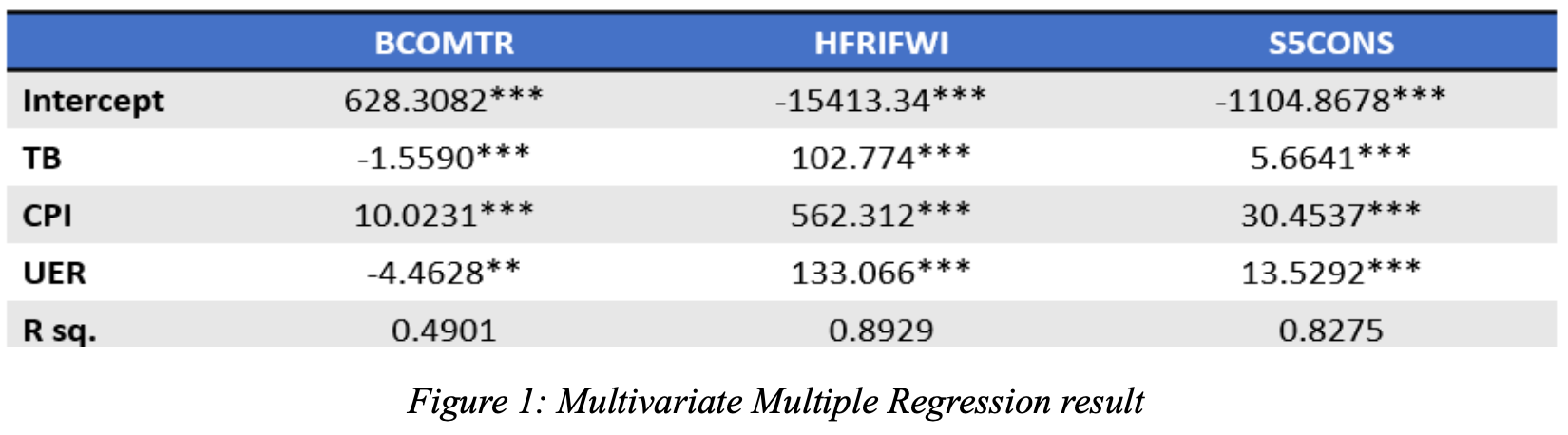
The methodologies employed range from statistical to machine learning models, aiming to thoroughly analyze market dynamics. The multivariate multiple regression, for instance, was applied to identify structural breaks and significant shifts in relationships between variables. However, this method did not yield desired results and led to the exploration of other models. The study then incorporated time-series modeling to detect anomalies, identifying irregular patterns or outliers in the data. For example, the Time-Series Plot of the S&P 500 consumer staples index (Figure 4) shows these patterns.
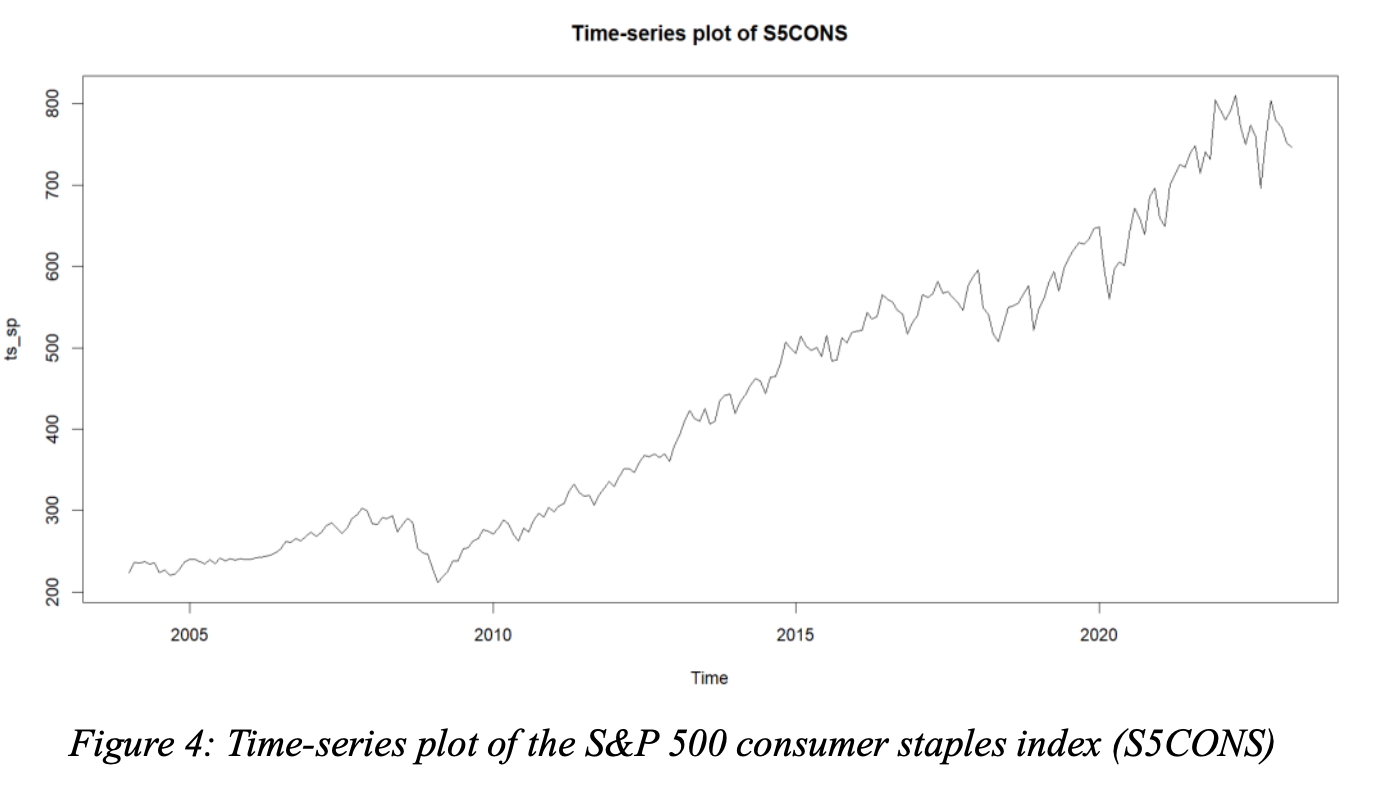
The Random Forest Model proved particularly effective for identifying periods of significant uncertainty. This approach was useful in identifying change points that indicate alterations in market behavior. The confusion matrix (Figure 15) shows the model's effectiveness in forecasting uncertainty levels using CPI and unemployment rate variables, which achieved nearly 97% accuracy. This level of precision was particularly obvious when recognizing low uncertainty levels. The red-shaded sections in Figure 14 represent periods of considerable uncertainty, which correspond to big price surges shown in the time series analysis.
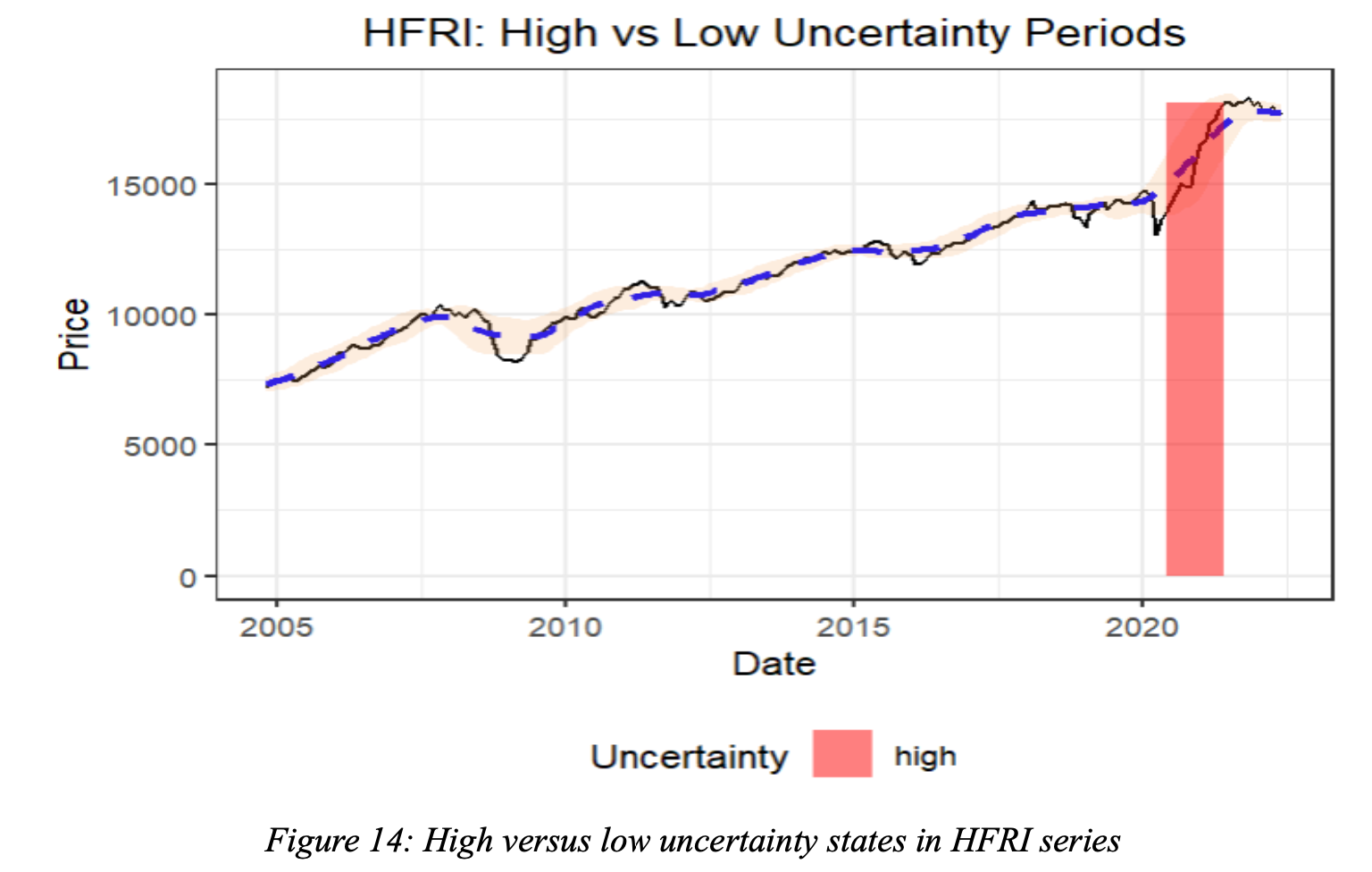
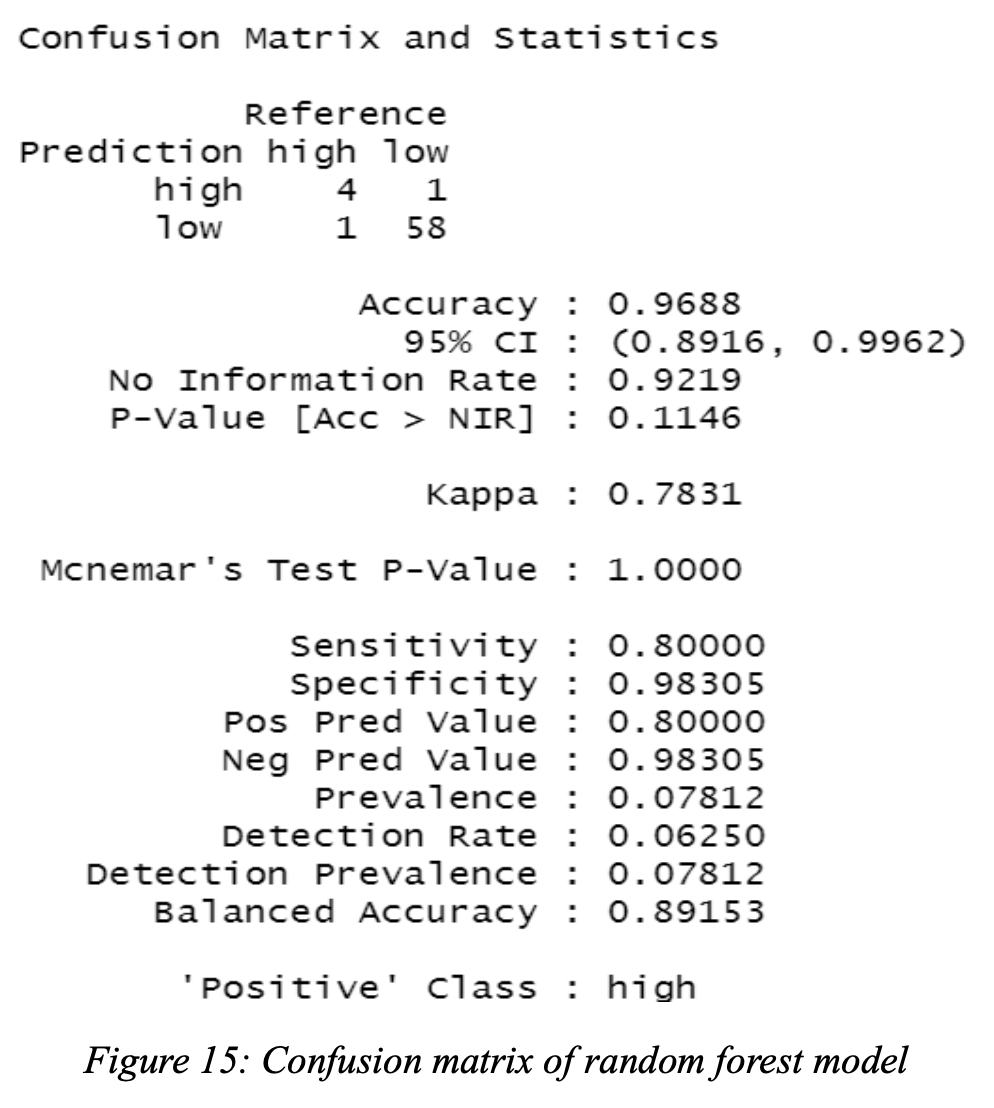
The research also included techniques like Markov Regime Switching (MRS), Hidden Markov Models (HMM), and Gaussian Mixture Models (GMM) as alternative ways to be able to detect various market regimes. These models have the benefit of recording regime transitions and offering insights into the specific statistical features for each regime. For example, when analyzing the S&P 500 Staples Index data insights were revealed regarding the economy's general health along with recognized patterns in consumer purchasing. The research also compared the CPI to SPY Last Price (Figure 14) to better understand how inflation and economic circumstances may affect consumer behavior.
Results
The research reveals many important facts in regards to market regime shifts, demonstrating transitions between different states such as bull, bear, stagnation, and recession. These insights are crucial for understanding market stability, volatility, and how economic data affects market patterns.
1) Impact of Economic Indicators: The reserach looks at how government bonds, inflation, and unemployment rates affect commodities, hedge funds, and the S&P 500 index. The regression results in Figure 1 demonstrate that all of the independent variables are statistically significant, indicating a strong relationship with changes in the dependent variables. The residuals analysis (Figure 2) reveals that, while the model's R-squared value for the hedge fund index and the S&P 500 consumer staples index is satisfactory, its performance is not without limitations.
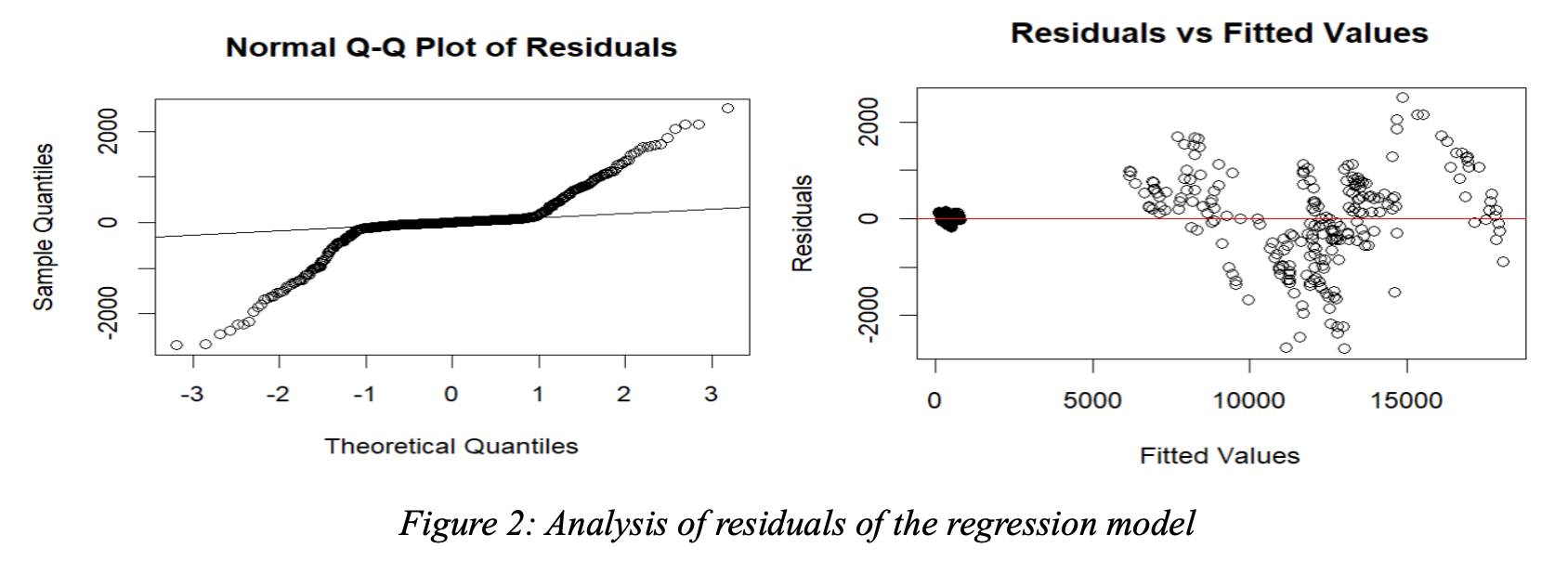
2) Random Forest Model Analysis: Using the random forest model to forecast high and low uncertainty states within the index using CPI and unemployment rate variables yielded a substantial variance explanation of 96.75% (Figure 13a). This high score indicates the model's resilience, particularly in recognizing periods of significant uncertainty. The variable significance measures (Figure 13b) indicated treasury bonds as an important predictive variable in the hedge fund index.
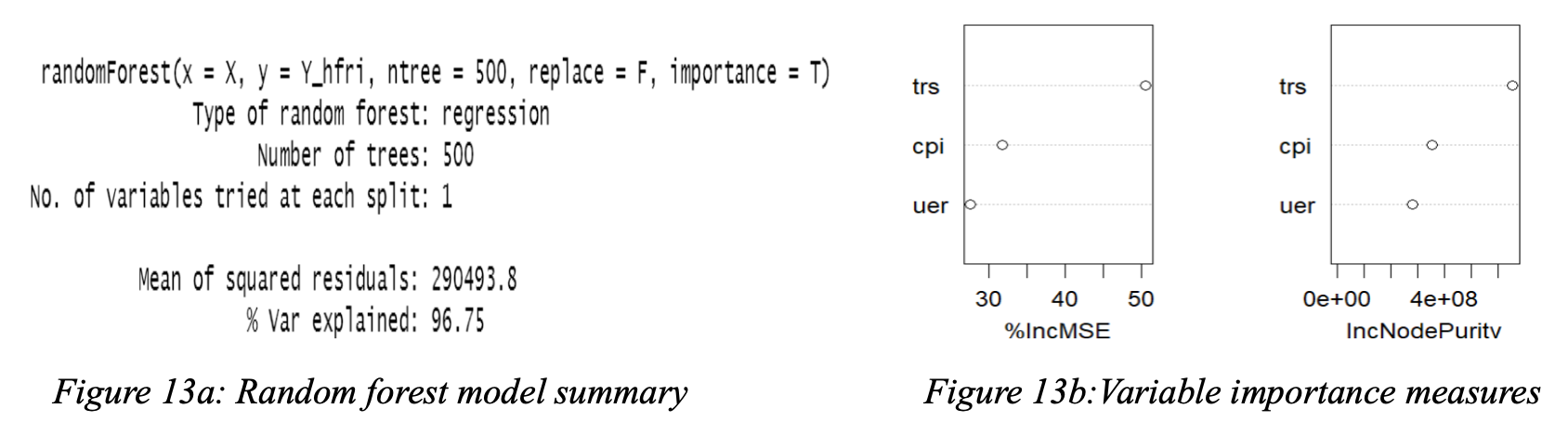
3) Time-Series Analysis and Anomaly Detection: The time-series modeling of the S&P 500 consumer staples index (Figure 4) initially showed no discernible patterns. However, further tests indicated departures from normal distribution and the presence of autocorrelations. Anomalies detected in the S&P 500 consumer staples index series indicate unusual market behavior or unforeseen events impacting the index.
4) Markov Regime Switching and Regime Transitions: The Markov Regime Switching analysis identified multiple regime shifts in the S&P 500, signaling significant transitions in market states. The study of the S&P 500 Staples data provided insights into consumer spending and the overall health of the economy. The analysis of regime transitions includes examining the duration and frequency of regime switches, which informs about the market's stability and volatility over time.
Conclusion
The research concludes with a detailed analysis that emphasizes both the intricacies of market dynamics and the effectiveness of regime-based asset allocation techniques. The study's detailed results emphasize the importance of using advanced statistical models such as the Markov Switching Model (MSM), Hidden Markov Model (HMM), and Gaussian Mixture Model (GMM) to gain a thorough understanding of market behaviors and improve the ability to predict market shifts. The combination of several approaches, including the insightful Random Forest Model (Figures 13a and 13b), illustrates their ability to properly foresee market behaviors and identify regime transitions.
Furthermore, the analysis supports the idea that regime-based asset allocation is a good way to manage systemic risks and optimize portfolio performance. By adjusting investment methods to contemporary market conditions, the study emphasizes the need of flexibility in investment decision-making. This strategy not only gives significant insights into market dynamics, but it also provides a solid foundation for responding to changes in the financial environment at the portfolio level, with the goal of outperforming more static strategies over time. Thus, the study is a critical resource for understanding the complexities of financial markets and the strategic value of regime-aware asset allocation in investment management.