Overview
Liquidity is one of the crucial factors in economy which reflects smooth operation of the market. In a liquid market, traders are able to transact large quantities of security quickly with minimal trading cost and price impact. Liquidity can measure different market characteristics such as trading time, tightness, depth, and resiliency. In the past few years, significant amount of literature try to shed light on the critical role of liquidity in finance. For instance, Researchers analyze the effect of liquidity in capital asset pricing model (CAPM) . The main issue with liquidity is that it is not directly observable in a market. Liquidity is easy to feel but hard to define. There are over 65 different measures of liquidity studied in literature. Since liquidity is a multi-dimension variable, defining a universal liquidity measure becomes a challenging problem. Therefore, despite liquidity’s importance, problems in quantifying liquidity still exists and defining a globally accepted measure for liquidity is an active area of research. Furthermore, most of the existing liquidity measures have been developed using monthly/daily (low frequency) data. However, today it is high frequency trading (HFT). Recently, HFT has become more popular in different areas of research specially the ones related to algorithmic trading (AT). Also, it is studied in literature that news sentiment can affect financial market and in some scenarios, it can lead to liquidity crisis. Therefore, analyzing liquidity behavior during a financial event is an interesting research topic that has drawn the attention of researchers recently.
We analyze commonality and idiosyncrasy pattern among existing liquidity measures using high frequency data. We use correlation to quantify similarity between liquidity measures. The following is an example of a correlation matrix calculated for some liquidity measures. Dark blue and red circles represent positive and negative correlation respectively. The color intensity is proportional to the correlation coefficient. As you can see, there are some liquidity measures that are highly correlated to each other.
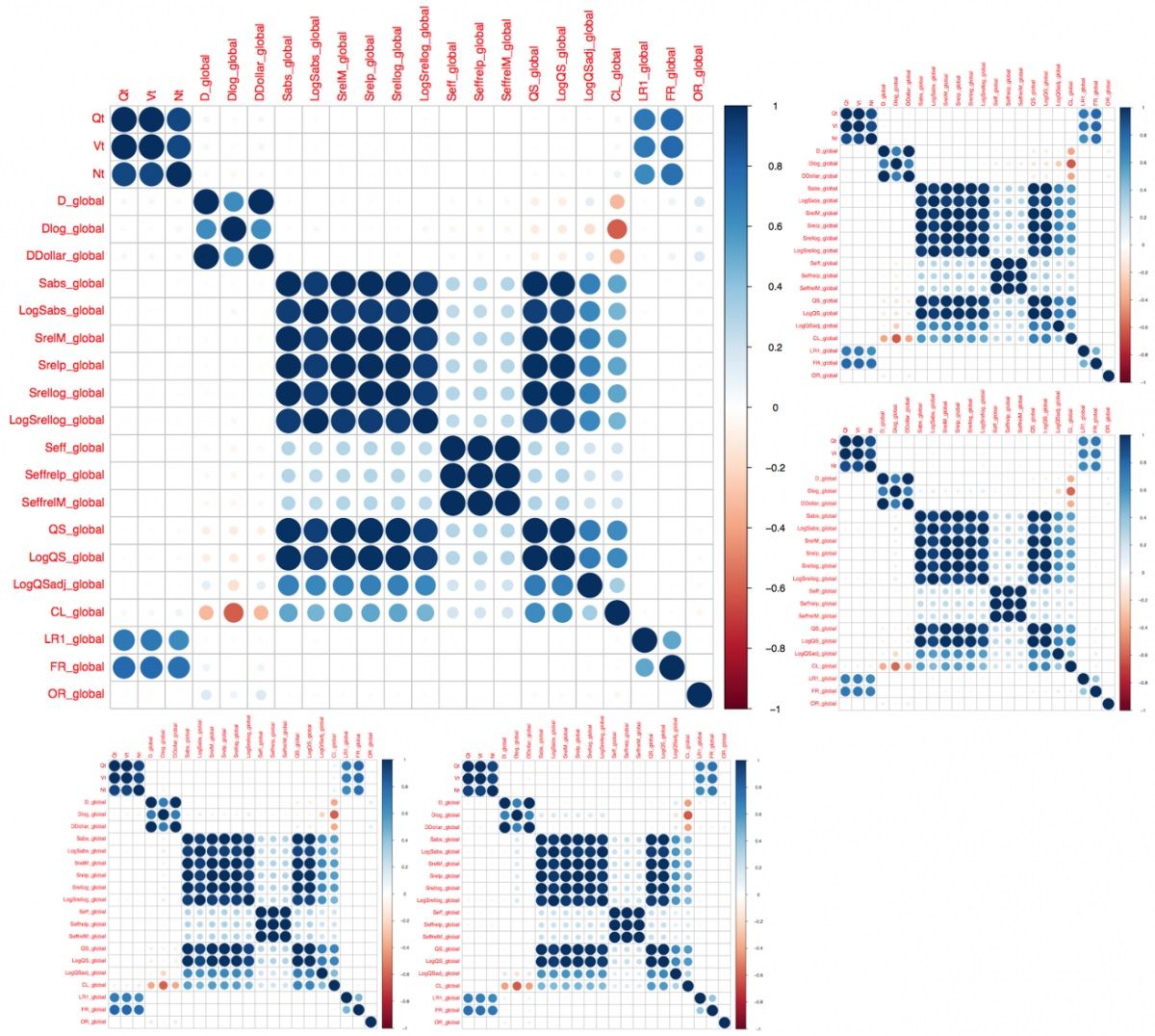
The results from correlation analysis can be used to develop a hierarchical clustering algorithm to classify liquidity measures into different groups. This approach can help researchers to identify set of liquidity measures that are providing same kind of information regarding market liquidity.
Research Topics:
- Developing a new liquidity index that can capture most of the market features.
- Developing a forecast system for financial security return by considering liquidity risk using convolution neural network (Deep Learning algorithm).
- Analyzing the effect of different liquidity measures in asset return.
- Liquidity and ETF return, event study: Brexit.
- Analyzing behavior (distribution change) of liquidity measures during financial events.
- Liquidity analysis in limit order book.
Publications:
1) A.Salighehdar, Y.Liu, D.Bozdog, I.Florescu " Cluster analysis of liquidity measures in a stock market using high frequency data", The 2017 International Conference on Management, Leadership and Business Intelligence (ICMLBI '17).
2) A.Salighehdar, Y.Liu, D.Bozdog, I.Florescu " Cluster analysis of liquidity measures in a stock market using high frequency data", Journal of Management Science and Business Intelligence (JMSBI) (under review)
Working Papers:
1) D.Mago, A.Salighehdar, M.Parekh, D.Bozdog, I.Florescu, “Market liquidity and asset return, event study: Brexit” , 2017 IEEE Symposium on Computational Intelligence for Financial Engineering & Economics (IEEE CIFEr'17)
2) M.Kong, A. Salighehdar, D.Bozdog, I.Florescu, “Distribution analysis for liquidity measures during Brexit” , 2017 IEEE Symposium on Computational Intelligence for Financial Engineering & Economics (IEEE CIFEr'17)
Presentations:
1) A.Salighehdar, Y.Liu, D.Bozdog, I.Florescu " Cluster analysis of liquidity measures in a stock market " , The 6th Annual High Frequency Finance and Data Analytics, October 29 - 31, 2015 (https://kolmogorov.fsc.stevens.edu/hff_conference2015/index.php/annual-frequency-conference/abstract-of-talks/50-amin-salighehdar-cluster-analysis-of-liquidity-measures-in-stock-market).
2) A.Salighehdar, Y.Liu, D.Bozdog, I.Florescu " Cluster analysis of liquidity measures in a stock market ", Graduate Research Conference at Stevens Institute of Technology, Feb 2016, Best Paper Presenattion Award from Dr. Constantin Chassapis (Vice Provost for Academics).
Researchers:
- Amin Salighehdar, Ph.D. Candidate in Financial Engineering (asalighe@stevens.edu)
- Dr. Ionut Florescu, Research Associate Professor in Department of Financial Engineering, Financial Systems Lab Director (ifloresc@stevens.edu)
- Dr. Dragos Bozdog, Teaching Associate Professor, Deputy Director of the Hanlon Financial Systems Lab (dragos.bozdog@stevens.edu)
Former Researchers:
1) Damini Mago, Mansi Parekh, Master studnet in Financial Engineering, FE800 Project, Spring 2017
2) Mingyuan Kong, Master studnet in Financial Engineering, FE 900, Spring 2017
3) Hongmin Chen, Shujie Zhang, Master studnet in Financial Engineering, FE800 Project, Spring 2016
4) Bo Shen, Yazhou Wu, Xuechao Qin, Master studnet in Financial Engineering, FE800 Project, Spring 2016